With the recent release of Fiix Asset Risk Predictor (ARP) I thought it would be a good time to talk a bit more about predictive maintenance (PdM). What is it? How is it different from other maintenance strategies, like condition-based maintenance? And when is predictive maintenance right for you? Let’s take a closer look at this often-misunderstood topic.
What is predictive maintenance?
Predictive maintenance (PdM) is a technique that uses data analysis tools and techniques to detect anomalies in your operation and possible defects in equipment and processes so you can fix them before they result in failure.
In other words, PdM attempts to see into the future so you can act to prevent failures before they happen. This proactive approach (the opposite of reactive!) is the essence of PdM. And the result isn’t just less downtime, it’s less waste in general. To see why, let’s place PdM in the context of other maintenance strategies using the simple example of a conveyor belt.
Reactive maintenance | The conveyor breaks down with no plan in place to fix it |
Run to fail maintenance | The conveyor is allowed to run until it breaks down with a plan to fix it |
Corrective maintenance | A technician notices that a part on the conveyor is misaligned during their weekly inspection and realigns it |
Routine maintenance | A machine operator inspects the conveyor before their shift to ensure it’s safe and free from obvious defects |
Preventive maintenance (Time) | The conveyor is inspected for potential failure every 10 days |
Preventive maintenance (Usage) | The conveyor is inspected for potential failure every five production cycles |
Condition-based maintenance | Maintenance is scheduled on the conveyor when vibration crosses a certain threshold |
Predictive maintenance | Software says that failure-level vibration on the conveyor belt will occur in 30 days |
Each strategy has its pros and cons, which we cover in-depth in The essential guide to choosing a maintenance strategy for your assets.
For our purposes, notice how approaches such as reactive and run-to-fail maintenance underutilize resources, resulting in downtime. Failures that could’ve been prevented with a simple inspection or spare part are simply allowed to happen. On the other hand, in approaches like routine and preventive maintenance, resources are consumed regardless of whether they’re truly needed. A worker may waste time inspecting a perfectly functioning machine or waste parts servicing it just because a time or usage counter said to. Downtime may be minimized, but resources are definitely overutilized.
Condition-based maintenance is a step in the right direction, but it often catches failures just as they’re happening or after they’ve happened. As a result, at least some downtime is still unavoidable (and of course, downtime is a form of waste). But with predictive maintenance, you have the insights you need to expend resources only when and where needed to prevent failures. That kind of precise targeting means less underutilization and less overutilization. So predictive maintenance isn’t just more proactive, it’s more efficient.
Peak maintenance maturity
We’ve talked a lot about the maintenance maturity journey in other posts. You may have noticed that we like to rank maintenance strategies from ‘operational’ to ‘strategic.’
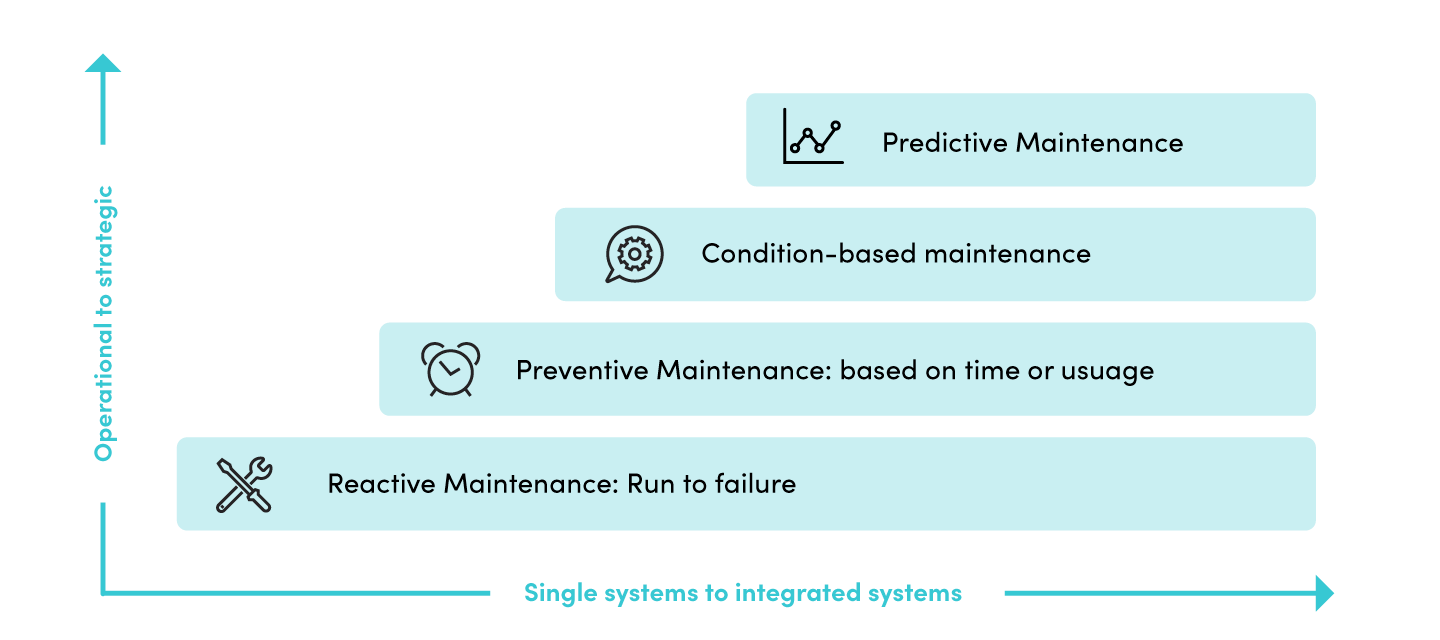
The increased resource efficiency of PdM is a great example of what we mean by ‘strategic.’ The focus isn’t only on fixing equipment, but optimizing the time, money, and energy going into that. Let’s complete this picture of ‘strategic’ maintenance by looking at some of the specific ways PdM can increase efficiency and benefit maintenance teams.
The benefits of predictive maintenance
In a sense, predictive maintenance is a form of condition-based maintenance: They’re both based on using data from your machines and sensors to drive maintenance decisions. That’s why I think it will be helpful to explain the unique benefits of PdM by comparing it to traditional condition-based maintenance (CBM). Of course, not all PdM solutions are created equally, so I’m going to be speaking specifically about Fiix Asset Risk Predictor (ARP) in what follows. Still, much of this holds true for PdM in general.
Compared to condition-based maintenance, predictive maintenance offers several clear advantages:
-
Automated learning: Unlike traditional condition-based monitoring where thresholds are manually set based on expert knowledge, data analysis or generic OEM guidelines, Asset Risk Predictor eliminates the need to manually set up thresholds and uses machine learning algorithms to learn what is normal for each asset automatically (after a learning period of about one week). This also means ARP can adapt to your specific recipes, processes, and operating conditions automatically, leading to more accurate predictions.
-
Intelligent anomaly detection: While traditional condition-based monitoring might trigger false alarms due to small deviations or spikes in sensor readings, the ARP algorithm is intelligent enough to ignore these minor anomalies that are not indicative of a problem.
-
Complex problem detection: Traditional condition-based monitoring often relies on simple thresholds that may not be sufficient to detect complex problems that involve a gradual change in condition over time or correlations between multiple data points. Asset Risk Predictor, however, can identify these complex patterns, allowing for earlier detection of potential issues.
-
Advanced detection: Unlike traditional condition-based monitoring, which tends to focus on current conditions, ARP includes next-day prediction capabilities (often spotting problems days or more in advance). This allows potential issues to be addressed well before they cause a failure.
-
Reliability metrics and predictions: Given failure codes from PLCs, Asset Risk Predictor can calculate reliability metrics, which are key indicators of an asset's probability of performing its intended function without failure over a specified period. By analyzing these metrics, Asset Risk Predictor can predict not only the risk of failure but also other aspects of asset performance, such as the expected remaining useful life of the asset.
All of these features increase your ability to determine when and where asset failure is likely to occur, so you can take early action and solve the problem as efficiently as possible.
The freedom to experiment
One of the less talked-about benefits of predictive maintenance is the freedom it gives teams to experiment. For example, if you’re changing out a part every month, maybe you want to change that to every six weeks and see what the impact is. More downtime? No change? How much money could you save per year by making that strategic change? Naturally, it can be nerve-wracking to tweak maintenance schedules on critical pieces of equipment. But with a solution like Fiix Asset Risk Predictor in place, you have a kind of insurance policy: If the scheduled maintenances become spaced too far apart, you still have a system in place to warn you of any impending failures and prevent unplanned downtime. In this way, PdM can give teams the confidence to change strategies and experiment with the critical assets that normally they’d be too timid to touch.
Note that in this case, PdM would be used on top of time-based scheduled maintenances (SM). This is a great example of how different strategies can be blended to achieve your goals. Nothing is mutually exclusive!
The cost vs. certainty trade-off
So predictive maintenance is sounding pretty good so far. But what about the downsides?
As a maintenance leader, you’re constantly faced with decisions: is it the optimal time to change this filter? Should I go to operations and shut this line down to do a major overhaul? As Jason Afara, a Fiix expert and former maintenance manager, told me, “It’s all about minimizing the amount of uncertainty in your work when you come in in the morning.” Unfortunately, certainty can be hard to come by. “In a perfect world, you would use predictive maintenance on every asset,” he says. “But it all depends on the resources you have. That’s the limiting factor for a maintenance leader, whether it’s labor constraints, budget constraints, or technical constraints.”
There’s the downside: predictive maintenance is generally more expensive to implement. That in turn means it’s usually only used on the most critical assets. (It’s no surprise then that determining asset criticality is an important step in choosing the right maintenance strategy. How do you determine asset criticality? Easy, we’ve created a free template for that).
That said, predictive maintenance these days is much cheaper and accessible than it used to be. As I noted in my last post, Fiix Asset Risk Predictor doesn’t require the Fortune 500-size budget or team of data scientists that some earlier solutions did. And while PdM is more expensive upfront, keep in mind that it can lead to cost-savings over time, especially in operations where downtime is costly.
Predictive maintenance programs have been shown to lead to a tenfold increase in ROI through:
-
25%-30% reduction in maintenance costs
-
70%-75% decrease of breakdowns
-
35%-45% reduction in downtime
Still, for less critical assets, CBM is often the economical choice, striking a balance between price and effectiveness. And there are ways to maximize the effectiveness of your CBM program, which we cover in this blog post.
Further reading
In our next article, we’ll dive deeper into predictive maintenance by breaking down the three most common PdM methodologies. Until then, check out our dedicated page on predictive maintenance strategies. It’s a fantastic resource that I don’t think enough people know about. We’ve also created A simple guide to building a predictive maintenance program, and it’s the perfect starting point for teams interested in PdM.
Till next time!
If you’re ready to make the leap to predictive maintenance with Fiix Asset Risk Predictor, now is the perfect time: until September 28th, we’re waiving the implementation fee ($8k value) and providing a free year of edge-to-cloud integration ($3k value) for new customers of ARP—a savings of over $11,000.
Update: This intro offer has ended but we still encourage you to get a conversation going with our experts on how you can level up your predictive maintenance strategy with ARP.